Compounded Iteration: How Machine Learning Saved Millions for Transport Companies
Brilliant ideas lead to success only if they are executed properly. Just having a brilliant idea doesn’t automatically ensure success unless you make it work. The ‘making your idea work’ is where a lot of companies get stuck. There are so many companies that have amazing concepts for bettering our lives with transformative services. However, they are stuck on the execution stage. And if not there, they are stuck on the innovation stage. But then again, there are those who do it right. There are the Apples, the Ubers, and the Amazons of the world. Do you want to know how they do it?
Consider the transportation industry. Transportation is no longer a support function, it is a necessity. The past year saw the highest amount of vehicle sales in most developed nations. The United States had more than 17.5 million car sales in 2016.
As Michelle Krebs, senior analyst at Autotrader, told The Washington Post, “Automakers accelerated incentives and promotions the last couple months of the year, sweetening the pot until the year closed. Their efforts paid off in higher-than-predicted December sales that [made] 2016 a record-setter.”
With all the cars coming into various parts of the world, how would the traffic scenario look in the near future? Traffic, in its considerations, is already blamed for almost every conceivable delay. How would traffic affect the demand for new cars? The world is gradually moving towards the Uber, Lyft, Ola, and Grab. Consumer movement is catching up with logistics transportation in some places and outperforming them in other places. But both sections of the transportation industry are set to balloon soon. Transportation is fast becoming a basic need.
What’s Next for the Transportation Industry? Machine Learning Enabled Route Optimization Solution!
Consumer expectations are galloping ahead of the speed of innovation. How would machine learning help in bridging this gap? Before we talk about that, what is ‘machine learning’? Machine learning is when a program within a solution of product learns from its usage history to make compounded iterations to better equip itself for future usage. You might have experienced this in advertising where you receive targeted ads as per your content consumption online. Machine learning is basically designing programs in a way that they gradually align themselves better with their pre-designated goals.
Machine learning helps transportation companies better understand routes and resource usage to better plan their operations. Route optimization software equipped with machine learning helps in transportation management. Effective route optimization solutions help direct the resource vehicles along the right track based on past traffic history on those particular routes. Real-time tracking helps them be more responsive. Real-time movement feedback adds on to the machine learning part of the program and helps the route optimization software suggest better iterated optimizations.
No two optimization plans are alike. Route planning that worked once wouldn’t necessarily work again as the circumstances have changed. In such situations, machine learning helps find patterns around the previous optimization plans to find a progressive planning curve which builds on itself.
How Does Machine Learning Help Reduce Costs? Effective Field Agent Management!
The basic movement costs for any transportation company are that of time and fuel. Both these costs are interwoven through a field agent management software which offers route planning and optimization solutions along with real-time tracking and feedback capture.
Field agents, in consumer transport, are a part of the overall service experience, adding to the overall requirement of real-time tracking.
Logistics transportation requires a much more comprehensive set of service level agreement (SLA) particulars depending on the shipments. All these factors have to be bought into consideration at the planning stage.
Machine learning enabled field agent management software helps in boosting resource utilization rates amongst the entire field force. Insufficient resource utilization, leading to idle vehicles, are also a big cost for such companies. Machine learning helps identify the locations, movement, and experience credentials for each resource. The experience credentials for vehicles are their load bearing capacities (in terms of logistics management) or aspirational or consumer satisfaction value (in consumer transportation). For field agents, these values reflect their on-field behavior pertaining to adherence with preset SLAs.
Time cost and resource cost is saved by helping companies direct the right resource to the right task and tracking their SLAs in real-time. With route optimization, bettered through the compounded iteration process of machine learning, traffic which can be avoided is avoided, saving time and fuels alike. With heightened resource utilization, the investments (vehicles) perform better, boosting the asset-to-profit ratio.
When the angle of market capture, under intense competition, arising from more vehicles on the streets and higher consumer satisfaction, is taken into consideration, the companies make money while saving money.
LogiNext has been aligning with many such transport companies helping them save millions in resource and optimization costs. The innovation curve is steep, but machine learning has made the journey much more manageable and achievable.
Cover image: Acrylic WiFi Heatmaps
192 1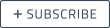
Pingback: Sonya_t | Pearltrees