What’s Going on in the Field? Be on Top of Ground-Level Realities
Someone wise once said, “There’s a difference between knowing the path and walking the path”. It’s true the quote, when it was said, had nothing to do with ground-level movement of field workforce for top CPG, maintenance service, cash management, distribution management companies. But the reality is that the distance between plans and execution mean that the companies are left wanting more and not able to figure out which bottleneck or efficiency drain to plug.
This is where we bring in machine learning-backed route planning scheduling in the picture. Let’s look at the issue as it stands right now.
The Field Workforce Planning-Execution Gap
Companies must perform tasks such as sales calls, client relationship building, maintenance services, supervising high-worth asset movement, etc. These tasks or work orders are planned manually. As there are many such tasks which need to be performed in a day, they must be put on a clear schedule for the field workforce to follow. This is where the manual intervention throws up issues. The ‘planning-execution gap’ begins here.
Suppose the plans are for a top CPG company which moves a high volume of consumer goods in the market. They normally deal with distributors and stockists which in turn deal with retailers. The distributors are visited by the company’s field sales professionals to record the required shipments. Further down the chain, the distributor’s field sales agents visit retailers for the latter’s replenishment requirements. Once the orders are placed, the goods are moved through proper last mile delivery optimization, which you might have read about in one of our other blogs.
Taking a step back to focus on the field sales agent’s activities, we see that their schedules are planned manually. This means that the manager has to account for multiple dynamic variables such as the preferred time windows for the outlets, when they were visited last (to avoid repeat visits), who visited them last (to avoid multiple agents visiting the same outlet for the same type of replenishment/product, also known as overlap or mirroring), the revenue or volume of orders coming in from different outlets (to keep a balanced and consistent supply of goods; and to manage a healthy lead time for all order delivery), etc.
Recommended Read: Are You Still Using Static Route Planning For Field Agent Management? Let’s Go Dynamic!
All these constraints add up. For a person to manually plan for all of them is difficult and not optimal. There are bound to be mistakes.
Top Problems Faced After Manual Field Workforce Planning |
|
Late visits | Repeat visits |
Missing the visit time window | Visiting limited number of outlets |
Long travel time between visits | Lesser revenue turnaround |
High cost of field movement | Lack of accountability |
This may lead to loss of client or retailer trust, affecting the relationship between the field agent and the retailer. It may also lead to loss of time and effort for the manager and the field agent, time and effort that could have been applied to more productive tasks.
The second half of the gap, the ‘execution’ is also often left to the active field agents. The field agents, without a clear planned schedule, decide on their own which outlets to visit first. They also decide which route to take to reach these outlets. The problem with this is:
Lack of Accountability: When field agents plan the sequence of their visits on their own, their activities are left unaccountable. What this means is that the manager won’t be able to figure why a particular agent is performing well or not. There would not be any way to utilize the learnings of positive on-ground activities or mistakes to be applied for future visits. The learning stays only with the agent and doesn’t benefit anyone else.
Lack of Consistency: As stated above, the learning from one ad-hoc activity can’t be accurately replicated for others. There would not be any consistent protocol or strategy which is followed by every agent. It would be a collection of random strategies (or no real strategy) followed by different agents.
Lack of Control: Without accountability and consistency, there isn’t much control over the on-ground activities for the supervising manager. Once the plan is made on the agents are dispatched, there won’t be a way to ‘track’ whether they actually follow the plan or just make up one of their own.
Lack of Optimal Movement: As we saw earlier, manual planning leads to inefficiencies. Whether it is the manager or the on-ground field agent, manual planning would never be optimal. There would always be a better way to do things, a more optimized method which would be constantly missed.
Or would it?
Filling the Planning-Execution Gap
LogiNext’s field workforce optimization solves all the above-mentioned problems and more. It’s machine learning-enabled planning engine pulls in all the constraints such as preferred time slot visits, avoiding repeat visits, avoiding overlap or mirroring, and also optimizing the service time or time spent at each outlet.
The planning engine gives the optimal schedule covering all constraints. It also maps the revenue and volume coming in from different outlets and assigns the field agent most-suited to maximize that potential. It also tracks live traffic to plan the shortest routes between the visits so that the field agent’s time is better spent with the client rather than just traveling.
These factors help bring down the cost of field workforce movement, increase the efficiency of their activities, boosts retailer/client-field agent relations, increases the order volume, increases the number of outlet visits covered per day while traveling lesser distance, and much more.
Coming to the other side of the equation, the live tracking of field agents gives complete control to the managers. The can know exactly where the agent is when they reach the desired outlet, how much time they spent there, and what their feedback/order is from the outlet. All this is done in real-time, so the manager has enough time to react to any eventuality or opportunity.
The field agents themselves are equipped with an intuitive app which makes their activities very simple. They can just mark themselves available at the start of their trip right from the app, eliminating the need to visit the sales office to mark the attendance. The time and location stamps validate the attendance. More importantly, they can just follow the schedules through the easy-interface map with clear directions and ETAs for each visit. They can record their feedback or response to each visit. They can even add tasks to themselves whenever required.
Recommended Read: Feature Snapshot! App-Driven Last Mile Delivery Optimization
Technology has caught up with us so that we don’t have to things manually which we don’t need to. We use instant messaging rather than telegrams because they are more convenient and efficient. The same is the case with optimization technology. It’s time to upgrade.
172 1
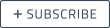
Pingback: Sonya_t | Pearltrees